
来源:深度学习与NLP
本文约2000字,建议阅读5分钟。
本文收集整理了一批基于Tensorflow实现的深度学习/机器学习的深度NLP模型。
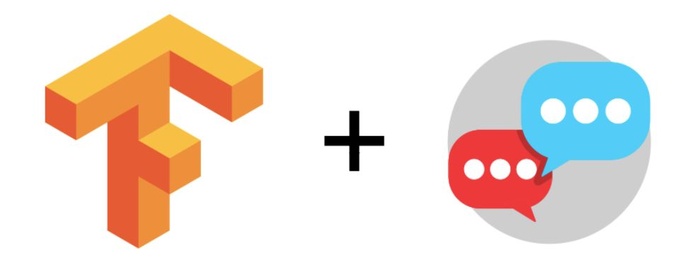
收集整理了一批基于Tensorflow实现的深度学习/机器学习的深度NLP模型。
基于Tensorflow的自然语言处理模型,为自然语言处理问题收集机器学习和Tensorflow深度学习模型,100%Jupeyter NoteBooks且内部代码极为简洁。
资源整理自网络,源地址:
https://github.com/huseinzol05
目录
Text classification
Chatbot
Neural Machine Translation
Embedded
Entity-Tagging
POS-Tagging
Dependency-Parser
Question-Answers
Supervised Summarization
Unsupervised Summarization
Stemming
Generator
Language detection
OCR (optical character recognition)
Speech to Text
Text to Speech
Text Similarity
Miscellaneous
Attention
目标
原始的实现稍微有点复杂,对于初学者来说有点难。所以我尝试将其中大部分内容简化,同时,还有很多论文的内容亟待实现,一步一步来。
内容
文本分类:
链接:
https://github.com/huseinzol05/NLP-Models-Tensorflow/tree/master/text-classification
1. Basic cell RNN
2. Bidirectional RNN
3. LSTM cell RNN
4. GRU cell RNN
5. LSTM RNN + Conv2D
6. K-max Conv1d
7. LSTM RNN + Conv1D + Highway
8. LSTM RNN with Attention
9. Neural Turing Machine
10. Seq2Seq
11. Bidirectional Transformers
12. Dynamic Memory Network
13. Residual Network using Atrous CNN + Bahdanau Attention
14. Transformer-XL
完整列表包含(66 notebooks)
聊天机器人:
链接:
https://github.com/huseinzol05/NLP-Models-Tensorflow/tree/master/chatbot
1. Seq2Seq-manual
2. Seq2Seq-API Greedy
3. Bidirectional Seq2Seq-manual
4. Bidirectional Seq2Seq-API Greedy
5. Bidirectional Seq2Seq-manual + backward Bahdanau + forward Luong
6. Bidirectional Seq2Seq-API + backward Bahdanau + forward Luong + Stack Bahdanau Luong Attention + Beam Decoder
7. Bytenet
8. Capsule layers + LSTM Seq2Seq-API + Luong Attention + Beam Decoder
9. End-to-End Memory Network
10. Attention is All you need
11. Transformer-XL + LSTM
12. GPT-2 + LSTM
完整列表包含(51 notebooks)
机器翻译(英语到越南语):
链接:
https://github.com/huseinzol05/NLP-ModelsTensorflow/tree/master/neural-machine-translation
1. Seq2Seq-manual
2. Seq2Seq-API Greedy
3. Bidirectional Seq2Seq-manual
4. Bidirectional Seq2Seq-API Greedy
5. Bidirectional Seq2Seq-manual + backward Bahdanau + forward Luong
6. Bidirectional Seq2Seq-API + backward Bahdanau + forward Luong + Stack Bahdanau Luong Attention + Beam Decoder
7. Bytenet
8. Capsule layers + LSTM Seq2Seq-API + Luong Attention + Beam Decoder
9. End-to-End Memory Network
10. Attention is All you need
完整列表包含(49 notebooks)
词向量:
链接:
https://github.com/huseinzol05/NLP-Models-Tensorflow/tree/master/embedded
1. Word Vector using CBOW sample softmax
2. Word Vector using CBOW noise contrastive estimation
3. Word Vector using skipgram sample softmax
4. Word Vector using skipgram noise contrastive estimation
5. Lda2Vec Tensorflow
6. Supervised Embedded
7. Triplet-loss + LSTM
8. LSTM Auto-Encoder
9. Batch-All Triplet-loss LSTM
10. Fast-text
11. ELMO (biLM)
词性标注:
链接:
https://github.com/huseinzol05/NLP-Models-Tensorflow/tree/master/pos-tagging
1. Bidirectional RNN + Bahdanau Attention + CRF
2. Bidirectional RNN + Luong Attention + CRF
3. Bidirectional RNN + CRF
实体识别:
链接:
https://github.com/huseinzol05/NLP-Models-Tensorflow/tree/master/entity-tagging
1. Bidirectional RNN + Bahdanau Attention + CRF
2. Bidirectional RNN + Luong Attention + CRF
3. Bidirectional RNN + CRF
4. Char Ngrams + Bidirectional RNN + Bahdanau Attention + CRF
5. Char Ngrams + Residual Network + Bahdanau Attention + CRF
依存分析:
链接:
https://github.com/huseinzol05/NLP-ModelsTensorflow/tree/master/dependency-parser
1. Bidirectional RNN + Bahdanau Attention + CRF
2. Bidirectional RNN + Luong Attention + CRF
3. Residual Network + Bahdanau Attention + CRF
4. Residual Network + Bahdanau Attention + Char Embedded + CRF
问答:
链接:
https://github.com/huseinzol05/NLP-Models-Tensorflow/tree/master/question-answer
1. End-to-End Memory Network + Basic cell
2. End-to-End Memory Network + GRU cell
3. End-to-End Memory Network + LSTM cell
词干抽取:
链接:
https://github.com/huseinzol05/NLP-Models-Tensorflow/tree/master/stemming
1. LSTM + Seq2Seq + Beam
2. GRU + Seq2Seq + Beam
3. LSTM + BiRNN + Seq2Seq + Beam
4. GRU + BiRNN + Seq2Seq + Beam
5. DNC + Seq2Seq + Greedy
有监督摘要抽取:
链接:
https://github.com/huseinzol05/NLP-Models-Tensorflow/tree/master/summarization
1. LSTM Seq2Seq using topic modelling
2. LSTM Seq2Seq + Luong Attention using topic modelling
3. LSTM Seq2Seq + Beam Decoder using topic modelling
4. LSTM Bidirectional + Luong Attention + Beam Decoder using topic modelling
5. LSTM Seq2Seq + Luong Attention + Pointer Generator
6. Bytenet
无监督摘要抽取:
链接:
https://github.com/huseinzol05/NLP-Models-Tensorflow/tree/master/unsupervised-summarization
1. Skip-thought Vector (unsupervised)
2. Residual Network using Atrous CNN (unsupervised)
3. Residual Network using Atrous CNN + Bahdanau Attention (unsupervised)
OCR (字符识别):
链接:
https://github.com/huseinzol05/NLP-Models-Tensorflow/tree/master/ocr
1. CNN + LSTM RNN
语音识别:
链接:
https://github.com/huseinzol05/NLP-Models-Tensorflow/tree/master/speech-to-text
1. Tacotron
2. Bidirectional RNN + Greedy CTC
3. Bidirectional RNN + Beam CTC
4. Seq2Seq + Bahdanau Attention + Beam CTC
5. Seq2Seq + Luong Attention + Beam CTC
6. Bidirectional RNN + Attention + Beam CTC
7. Wavenet
语音合成:
链接:
https://github.com/huseinzol05/NLP-Models-Tensorflow/tree/master/text-to-speech
1. Tacotron
2. Wavenet
3. Seq2Seq + Luong Attention
4. Seq2Seq + Bahdanau Attention
生成器:
链接:
https://github.com/huseinzol05/NLP-Models-Tensorflow/tree/master/generator
1. Character-wise RNN + LSTM
2. Character-wise RNN + Beam search
3. Character-wise RNN + LSTM + Embedding
4. Word-wise RNN + LSTM
5. Word-wise RNN + LSTM + Embedding
6. Character-wise + Seq2Seq + GRU
7. Word-wise + Seq2Seq + GRU
8. Character-wise RNN + LSTM + Bahdanau Attention
9. Character-wise RNN + LSTM + Luong Attention
语言检测:
链接:
https://github.com/huseinzol05/NLP-Models-Tensorflow/tree/master/language-detection
1. Fast-text Char N-Grams
文本相似性:
链接:
https://github.com/huseinzol05/NLP-Models-Tensorflow/tree/master/text-similarity
1. Character wise similarity + LSTM + Bidirectional
2. Word wise similarity + LSTM + Bidirectional
3. Character wise similarity Triplet loss + LSTM
4. Word wise similarity Triplet loss + LSTM
注意力机制:
链接:
https://github.com/huseinzol05/NLP-Models-Tensorflow/tree/master/attention
1. Bahdanau
2. Luong
3. Hierarchical
4. Additive
5. Soft
6. Attention-over-Attention
7. Bahdanau API
8. Luong API
其他:
链接:
https://github.com/huseinzol05/NLP-Models-Tensorflow/tree/master/misc
1. Attention heatmap on Bahdanau Attention
2. Attention heatmap on Luong Attention
非深度学习:
链接:
https://github.com/huseinzol05/NLP-Models-Tensorflow/tree/master/not-deep-learning
1. Markov chatbot
2. Decomposition summarization (3 notebooks)
编辑:王菁
校对:林亦霖
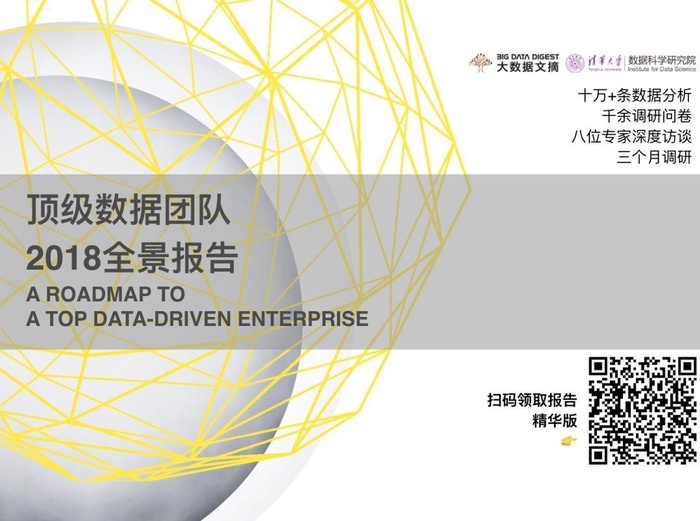
