importtensorflowastfprint(tf.__version__)atf.constant(2.0)print(a) #声明一个标量常量t_1tf.constan
import tensorflow as tf
print(tf.__version__)
a = tf.constant(2.0)
print(a)
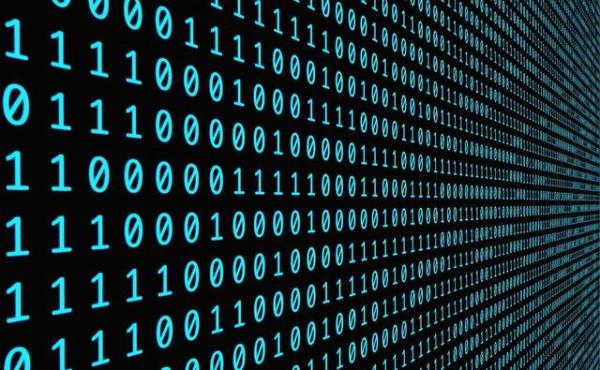
#声明一个标量常量
t_1 = tf.constant(2)
t_2 = tf.constant(2)
#常量相加
t_add = tf.add(t_1,t_2)
#一个形如一行三列的常量向量可以用如下代码声明
t_3 = tf.constant([4,3,2])
#定义一个形状为[M,N]的全0张量和全1张量
zeros = tf.zeros(shape=[3,3])
Ones= tf.ones(shape=[3,3])
#直接赋值初始化
import tensorflow as tf
#直接给变量赋值初始化
bias1 = tf.Variable(2)
#通过initial_value显示的赋值初始化
bias2 = tf.Variable(initial_value=3.)
#使用初始化函数初始化
a=tf.Variable(tf.zeros([2,1])) #将形状为[2,1]张量初始化为0
b=tf.Variable(tf.zeros_like(a)) #返回一个和给定tensor同样shape的tensor,其中的元素全部置0
c=tf.Variable(tf.ones([2,1])) #初始化为1
d=tf.Variable(tf.ones_like(a)) #将与a一个形状的张量初始化为1
e=tf.fill([2,3],4) #将指定形状的张量初始化为指定数值
import tensorflow as tf
a=tf.constant([[1.0,2.0],[3.0,4.0]])
print(a.shape)
print(a.dtype)
print(a.numpy())

Tensorflow的基础运算操作
import tensorflow as tf
print(tf.add(1,2)) #0维张量相加
print(tf.add([1,2],[3,4])) #一维张量相加
print(tf.matmul([[1,2,3]],[[4],[5],[6]])) #矩阵相乘
print(tf.square(5)) #计算5的平方
print(tf.pow(2,3)) #计算2的3次方
print(tf.square(2)+tf.square(3)) #也支持操作符重载
print(tf.reduce_sum([1,2,3])) #计算数值的和
print(tf.reduce_mean([1,2,3])) #计算均值
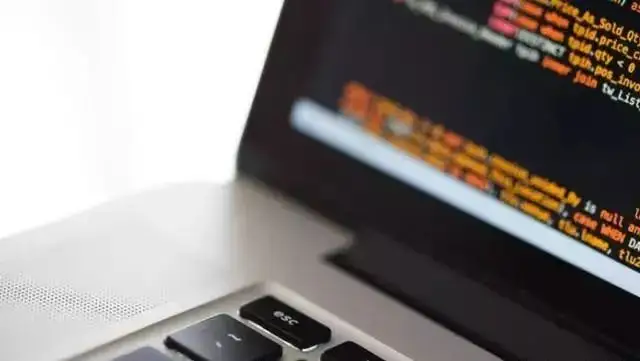
模型搭建时常用的Tensor操作
(1)取最大索引:tf.argmax
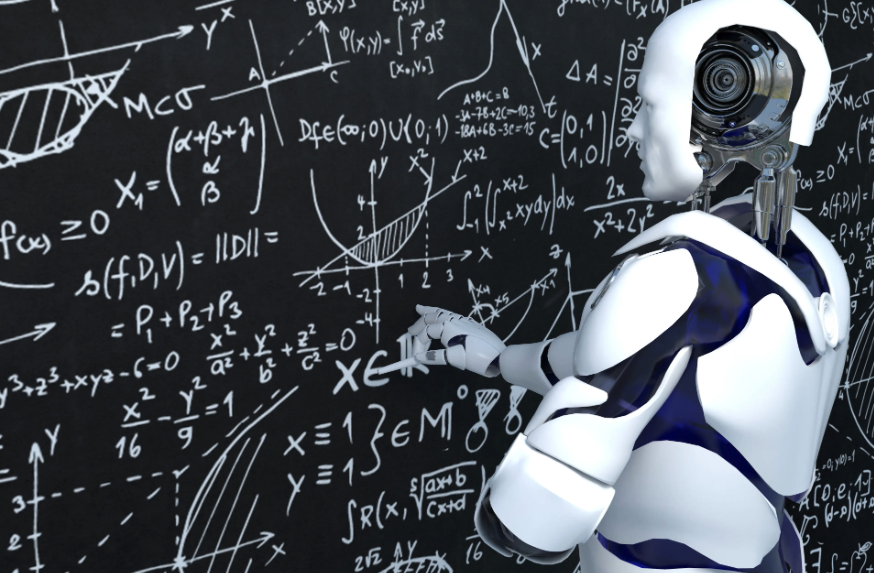
(2)扩张维度:tf.expand_dims

(3)张量拼接:tf.concat
x=[[1,2,3],[4,5,61],[7,8,9]]
y=[[2,3,4],[5,6,7],[8,9,10]]
z1=tf.concat([x,y],axis=0) #按照维度0拼接
z2=tf.concat([x,y],axis=1) #按照维度1拼接
print(z1,z2)

(4)形状变换:tf.reshape
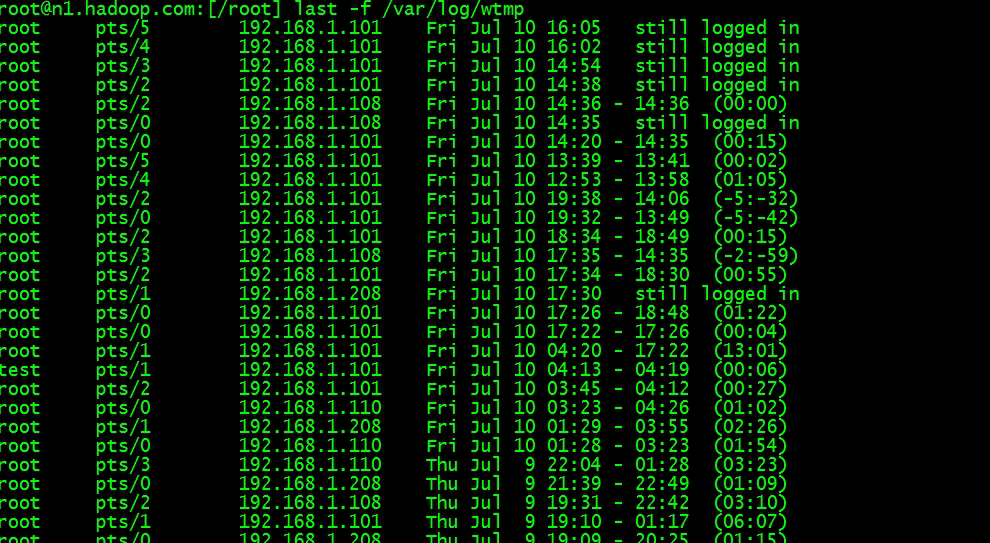
课后作业
import tensorflow as tf
from tensorflow import keras
import numpy as np
import matplotlib.pyplot as plt
fashion_mnist = keras.datasets.fashion_mnist
(train_images, train_labels), (test_images, test_labels) = fashion_mnist.load_data()
class_names = ['T-shirt/top', 'Trouser', 'Pullover', 'Dress', 'Coat',
'Sandal', 'Shirt', 'Sneaker', 'Bag', 'Ankle boot']
train_images.shape
len(train_labels)
train_labels
test_images.shape
len(test_labels)
plt.figure()
plt.imshow(train_images[0])
plt.colorbar()
plt.grid(False)
plt.show()
train_images = train_images / 255.0
test_images = test_images / 255.0
plt.figure(figsize=(10,10))
for i in range(25):
plt.subplot(5,5,i+1)
plt.xticks([])
plt.yticks([])
plt.grid(False)
plt.imshow(train_images[i], cmap=plt.cm.binary)
plt.xlabel(class_names[train_labels[i]])
plt.show()
model = keras.Sequential([
keras.layers.Flatten(input_shape=(28, 28)),
keras.layers.Dense(128, activation='relu'),
keras.layers.Dense(10)
])
model.compile(optimizer='adam',
loss=tf.keras.losses.SparseCategoricalCrossentropy(from_logits=True),
metrics=['accuracy'])
model.fit(train_images, train_labels, epochs=10)
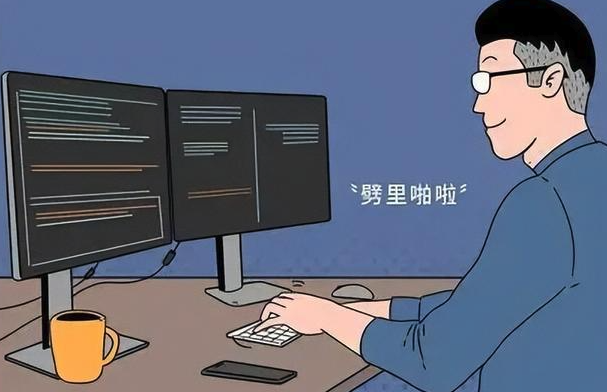

#模型对于全部 10 个类的预测
def plot_image(i, predictions_array, true_label, img):
predictions_array, true_label, img = predictions_array, true_label[i], img[i]
plt.grid(False)
plt.xticks([])
plt.yticks([])
plt.imshow(img, cmap=plt.cm.binary)
predicted_label = np.argmax(predictions_array)
if predicted_label == true_label:
color = 'blue'
else:
color = 'red'
plt.xlabel("{} {:2.0f}% ({})".format(class_names[predicted_label],
100*np.max(predictions_array),
class_names[true_label]),
color=color)
def plot_value_array(i, predictions_array, true_label):
predictions_array, true_label = predictions_array, true_label[i]
plt.grid(False)
plt.xticks(range(10))
plt.yticks([])
thisplot = plt.bar(range(10), predictions_array, color="#777777")
plt.ylim([0, 1])
predicted_label = np.argmax(predictions_array)
thisplot[predicted_label].set_color('red')
thisplot[true_label].set_color('blue')
i = 0
plt.figure(figsize=(6,3))
plt.subplot(1,2,1)
plot_image(i, predictions[i], test_labels, test_images)
plt.subplot(1,2,2)
plot_value_array(i, predictions[i], test_labels)
plt.show()
i = 12
plt.figure(figsize=(6,3))
plt.subplot(1,2,1)
plot_image(i, predictions[i], test_labels, test_images)
plt.subplot(1,2,2)
plot_value_array(i, predictions[i], test_labels)
plt.show()
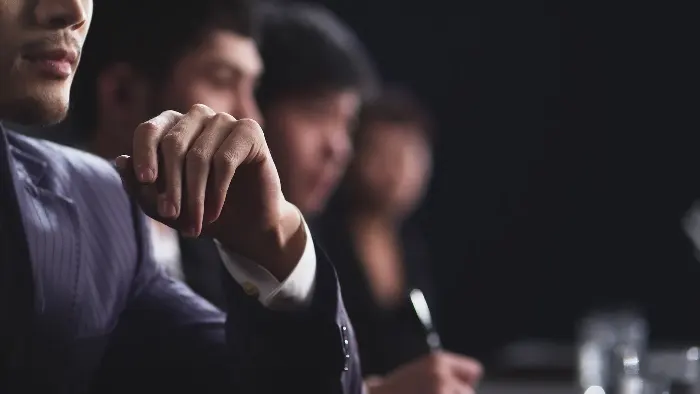