作者:路易公子_352 | 来源:互联网 | 2023-08-04 14:32
在医学领域,模型的可解释性尤为重要,需要有明确的结论证据支持,然后牵涉到神经网络模型,绕不开的就是黑盒观点。
业界做了很多尝试,发现一片不错的模型可解释介绍。这里依旧做要点记录,以免原链接失效。
原链接:https://www.jiqizhixin.com/articles/2019-10-30-9
1. 什么是模型的可解释性
模型解释的三个最重要的方面解释:
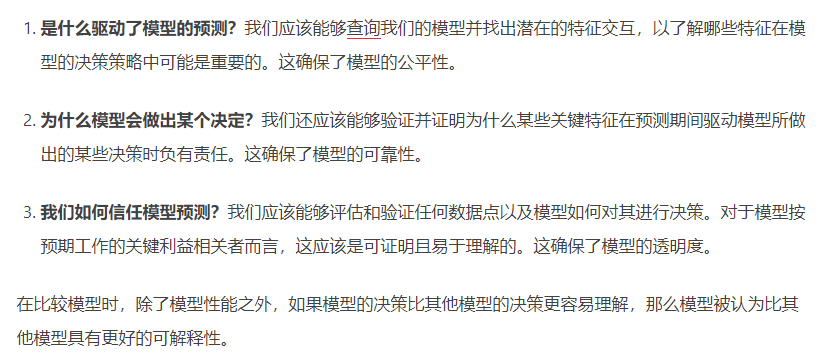
2. 可解释性的标准
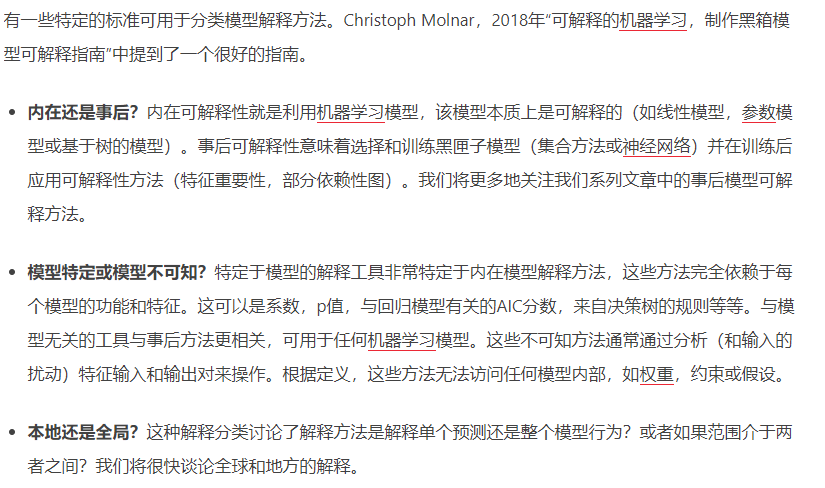
3.可解释性的作用
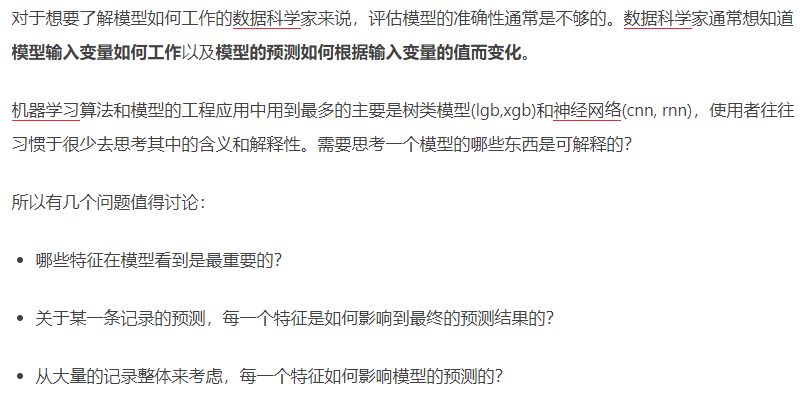
在医学领域,结果的可解释性是非常重要的。
4.解释性模型的方法:重点关注和神经网络相关的方法
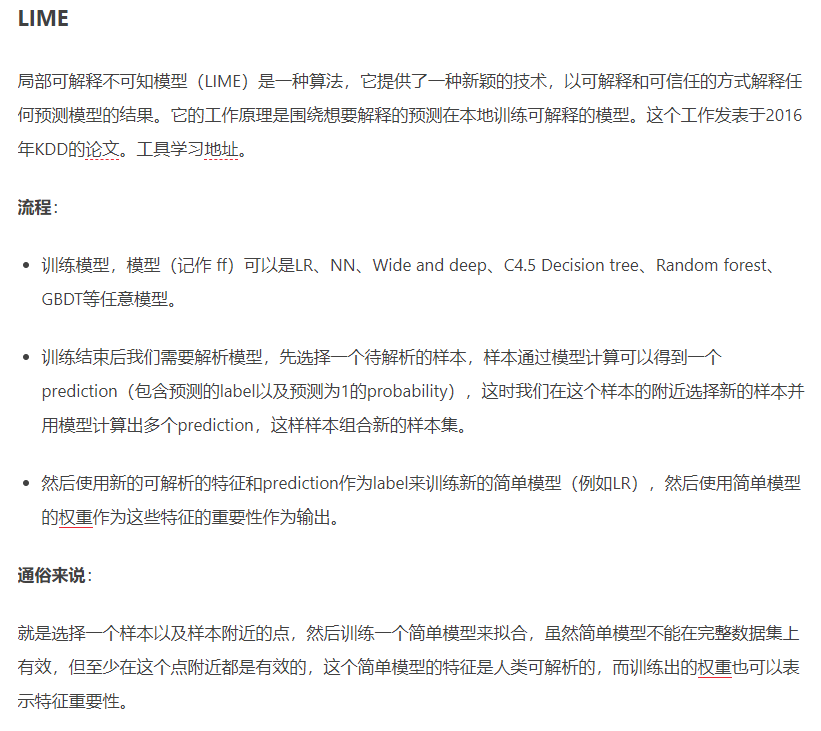
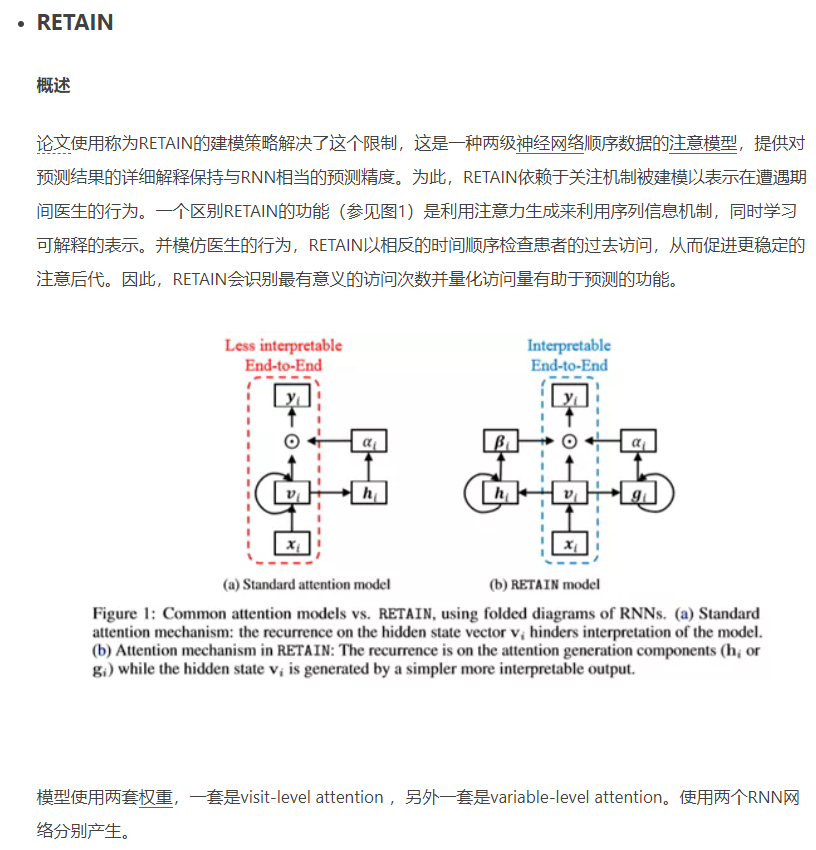
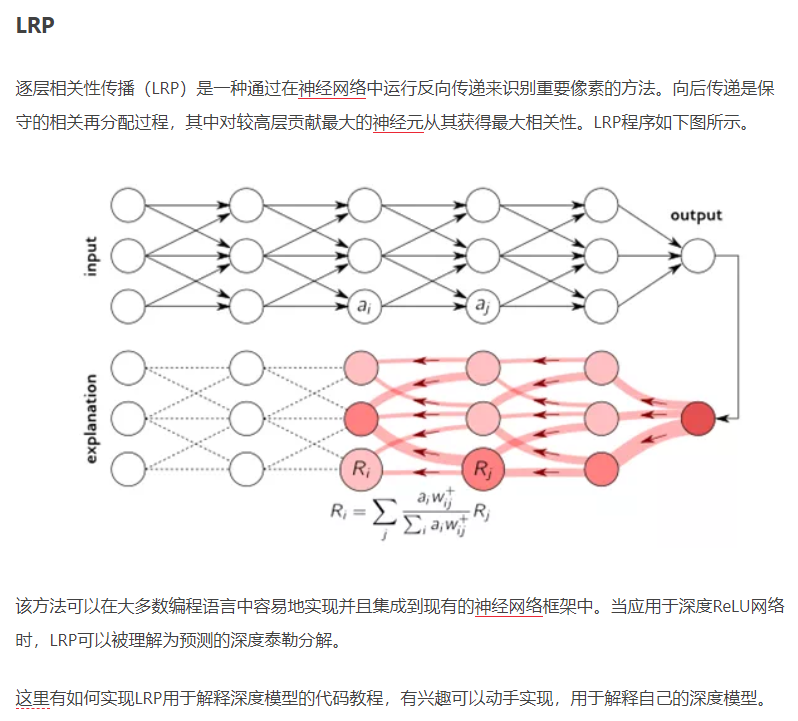
参考文献
-
Interpretable Machine Learning
https://christophm.github.io/interpretable-ml-book/pdp.html
-
Partial dependence ——集成树的可解析性
https://zhuanlan.zhihu.com/p/40356430
-
Machine Learning for Insights Challenge
https://zhuanlan.zhihu.com/p/45898896
-
https://yyqing.me/post/2018/2018-09-25-kaggle-model-insights
-
http://rstudio-pubs-static.s3.amazonaws.com/283647_c3ab1ccee95a403ebe3d276599a85ab8.html
-
《通向人类可理解、可解释的人工智能》
-
https://github.com/lopusz/awesome-interpretable-machine-learning
-
https://github.com/jphall663/awesome-machine-learning-interpretability
-
https://github.com/Henrilin28/awesome-Interpretable-ML
-
http://xiangruix.com/2018/07/31/lime/
-
https://www.jianshu.com/p/b52efa66154e
-
https://zhuanlan.zhihu.com/p/32891505
-
https://suensummit.github.io/intro-lime/#1
-
https://www.oreilly.com/learning/introduction-to-local-interpretable-model-agnostic-explanations-lime
-
https://github.com/slundberg/shap
-
http://km.oa.com/group/22630/articles/show/380452?kmref=search&from_page=1&no=1
以上介绍对探索贝叶斯方法的可解释性有重大参考意义。贝叶斯方法的可解释性,个人觉得有两方面:一是先验概念引入;二是超参最优化的稀疏解作用。具体有待实验证明。