作者:我爱麦兜李 | 来源:互联网 | 2023-08-01 12:52
原标题:TrafficPredict Trajectory Predictionfor Heterogeneous Traffic-Agents
作者:Yuexin Ma, Xinge Zhu, Sibo Zhang,Ruigang Yang, Wenping Wang, Dinesh Manocha
关键词:Spatial-Temporal, LSTM, Attention, TrajectoryPrediction
中文摘要:
为了在复杂的城市交通中安全有效地导航,自动驾驶车辆必须对周围的交通代理者(车辆、自行车、行人等)做出负责任的预测。探索不同交通代理者的运动模式,准确预测其未来轨迹,帮助自动驾驶车辆做出合理的导航决策,是一项具有挑战性和关键性的任务。为了解决这一问题,我们提出了一种基于LSTM模型的实时流量预测算法——TrafficPredict。我们的方法是使用个体层来学习每个个体的移动和交互模式,使用类别层来学习属于同一类型的个体之间的相似性,进而来提高预测的准确性。为了评估该模型的性能,我们收集了一个大城市不同条件和交通密度的轨迹数据集。数据集包括许多具有挑战性的场景,其中车辆、自行车和行人在彼此之间互相移动。我们在新数据集上评估了TrafficPredict算法的性能,并将其与之前的预测方法进行比较,结果表明它在轨迹预测方面的精度更高。
英文摘要:
To safely and efficiently navigate incomplex urban traffic, autonomous vehicles must make responsible predictions inrelation to surrounding traffic-agents (vehicles, bicycles, pedestrians, etc.).A challenging and critical task is to explore the movement patterns ofdifferent traffic-agents and predict their future trajectories accurately tohelp the autonomous vehicle make reasonable navigation decision. To solve thisproblem, we propose a long short-term memory-based (LSTM-based) realtimetraffic prediction algorithm, TrafficPredict. Our approach uses an instancelayer to learn instances’ movements and interactions and has a category layerto learn the similarities of instances belonging to the same type to refine theprediction. In order to evaluate its performance, we collected trajectorydatasets in a large city consisting of varying conditions and trafficdensities. The dataset includes many challenging scenarios where vehicles,bicycles, and pedestrians move among one another. We evaluate the performanceof TrafficPredict on our new dataset and highlight its higher accuracy fortrajectory prediction by comparing with prior prediction methods.
原文链接:https://arxiv.org/abs/1811.02146
文献总结:
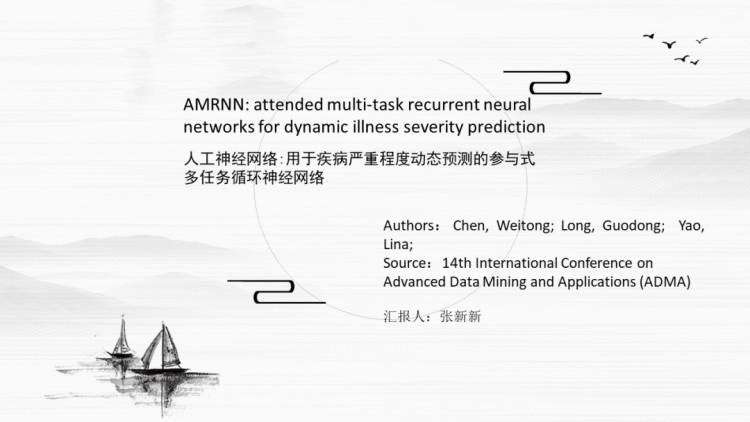
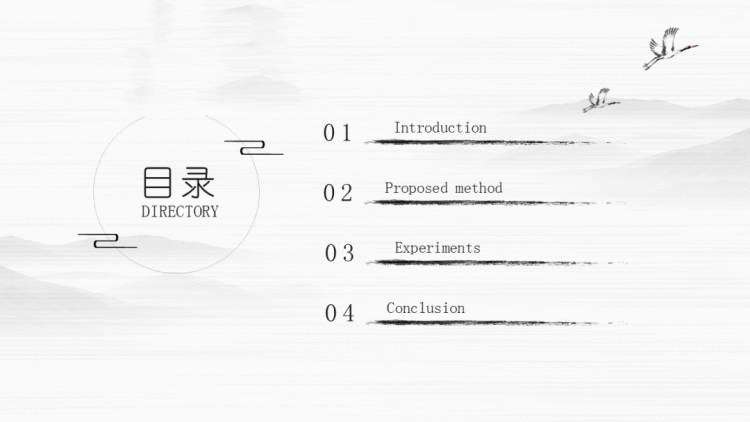
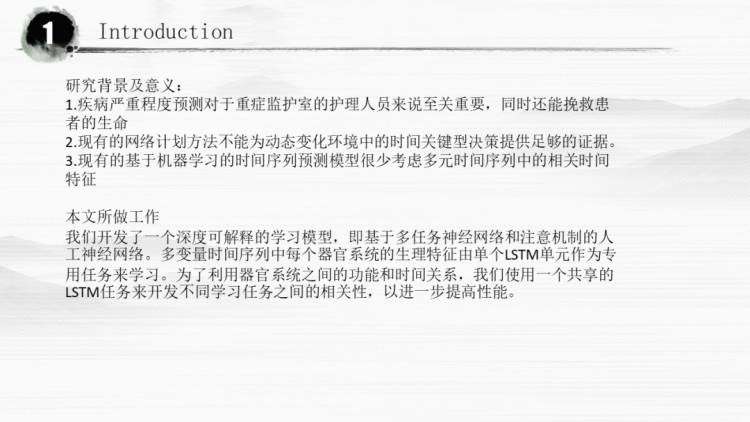
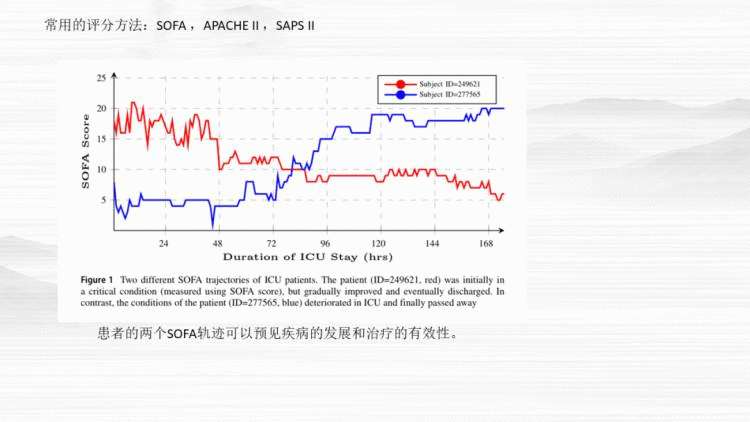
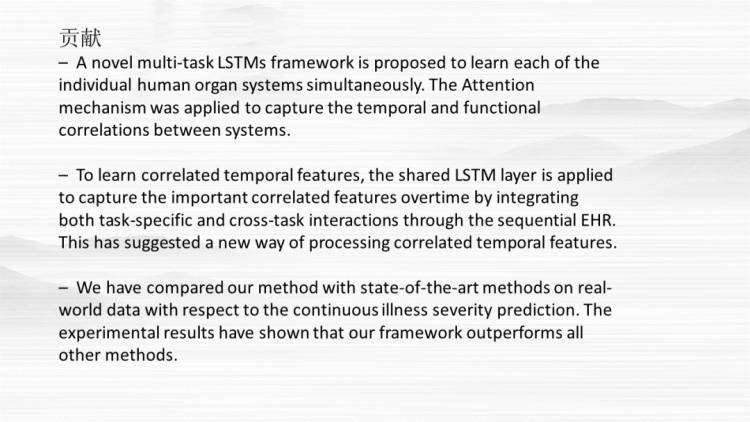
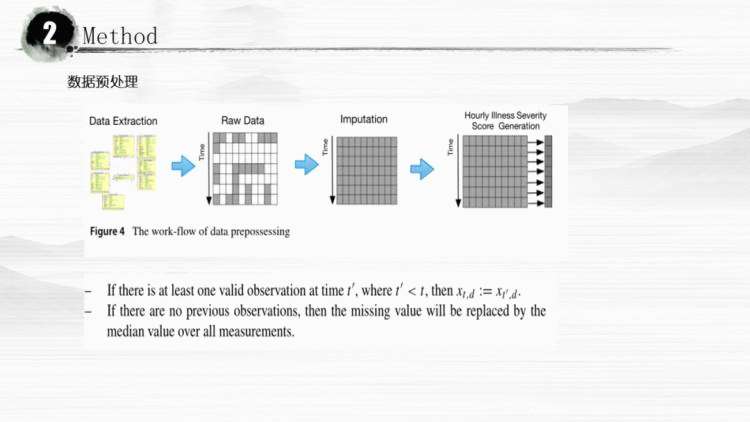
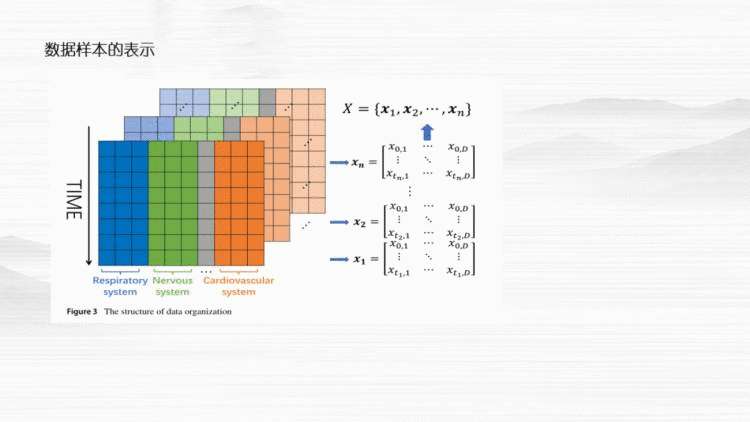
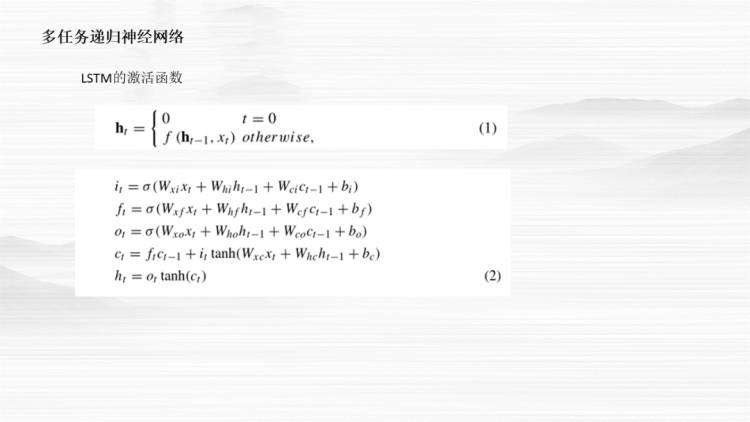
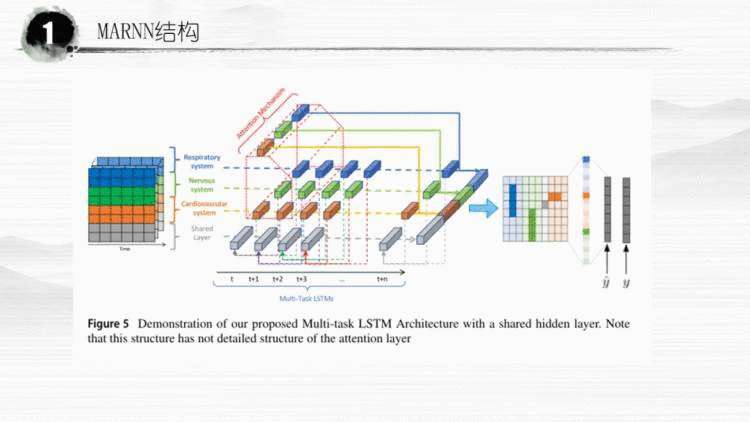
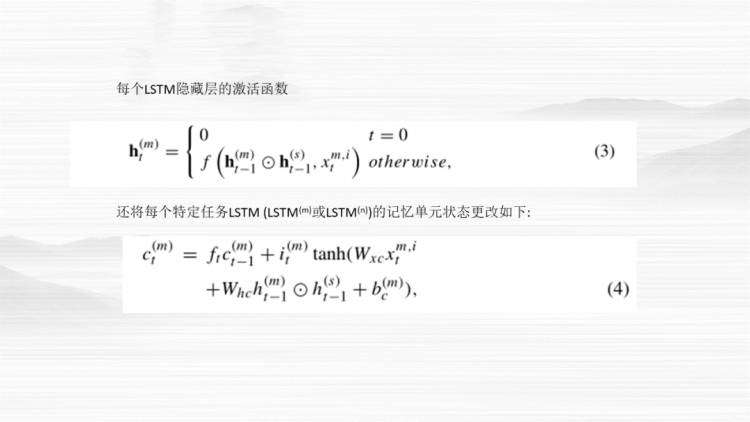
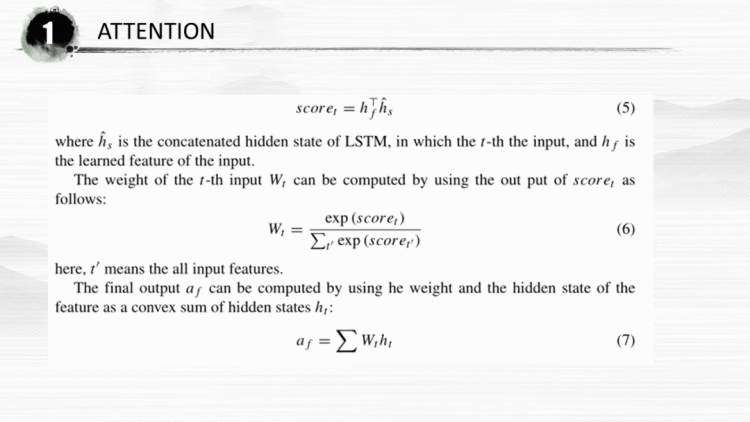
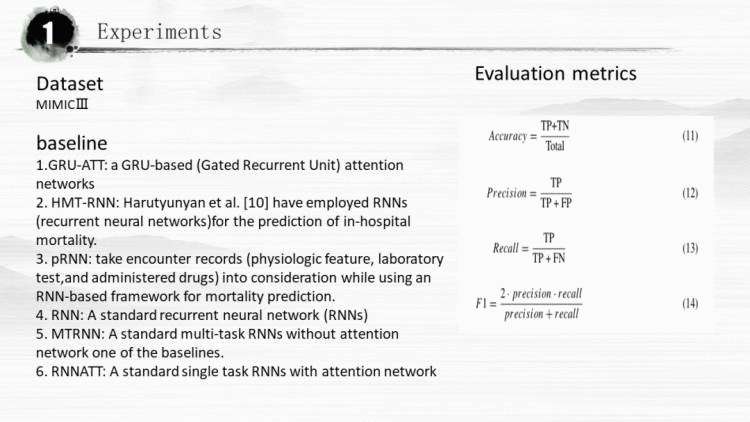
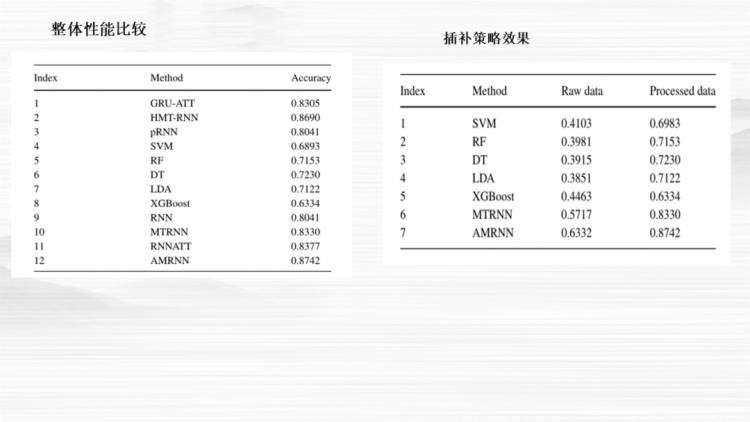
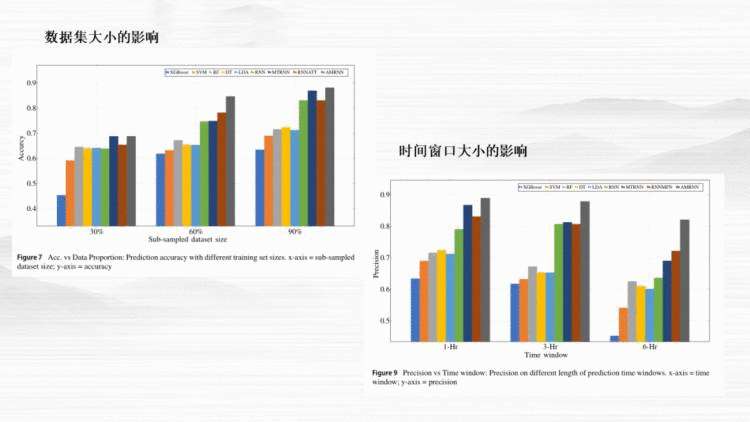
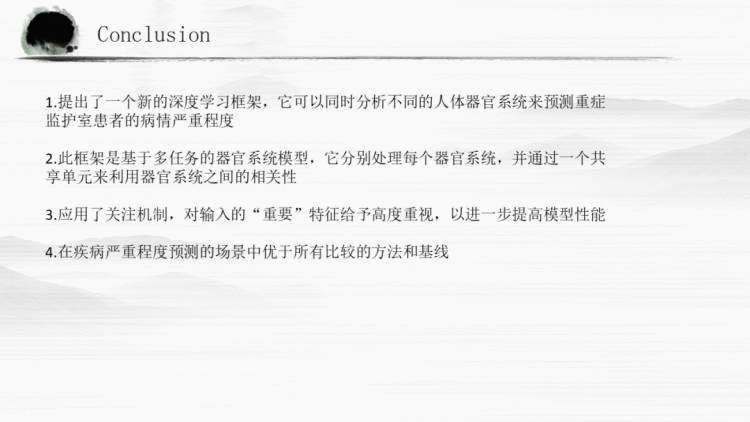